Fraud Detection Using Machine Learning in Banking
The financial landscape is evolving rapidly. With this evolution comes an increase in financial crimes, particularly fraud.
Financial institutions are constantly seeking ways to enhance their fraud detection and prevention mechanisms. Traditional methods, while effective to some extent, often fall short in the face of sophisticated fraudulent schemes.
Enter machine learning. This technology has emerged as a game-changer in the banking sector, particularly in fraud detection.
Machine learning algorithms can sift through vast volumes of transaction data, identifying patterns and anomalies indicative of fraudulent activities. This ability to learn from historical data and predict future frauds is revolutionising the way financial institutions approach fraud detection.
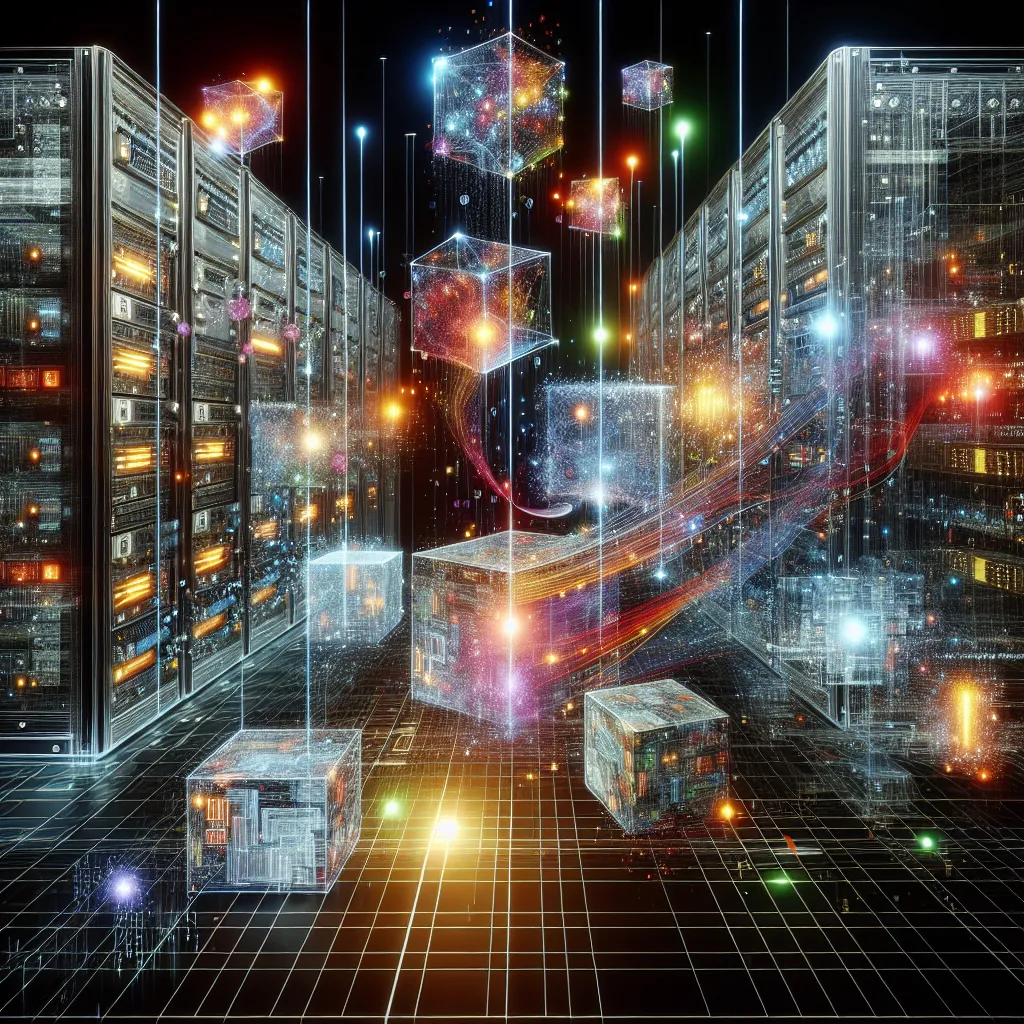
However, the implementation of machine learning in fraud detection is not without its challenges. Distinguishing between legitimate transactions and suspicious activity, ensuring data privacy, and maintaining regulatory compliance are just a few of the hurdles to overcome.
This article aims to provide a comprehensive overview of fraud detection using machine learning in banking. It will delve into the evolution of fraud detection, the role of machine learning, its implementation, and the challenges faced.
By the end, financial crime investigators and other professionals in the banking sector will gain valuable insights into this cutting-edge technology and its potential in enhancing their fraud detection strategies.
The Evolution of Fraud Detection in Banking
The banking sector has always been a prime target for fraudsters. Over the years, the methods used to commit fraud have evolved, becoming more complex and sophisticated.
In response, financial institutions have had to adapt their fraud detection systems. Traditional fraud detection methods relied heavily on rule-based systems and manual investigations. These systems were designed to flag transactions that met certain predefined criteria indicative of fraud.
However, as the volume of transactions increased with the advent of digital banking, these traditional systems began to show their limitations. They struggled to process the vast amounts of transaction data, leading to delays in fraud detection and prevention.
Moreover, rule-based systems were often unable to detect new types of fraud that did not fit into their predefined rules. This led to a high number of false negatives, where fraudulent transactions went undetected.
The need for a more effective solution led to the exploration of machine learning for fraud detection.
Traditional Fraud Detection vs. Machine Learning Approaches
Traditional fraud detection systems, while useful, often lacked the ability to adapt to new fraud patterns. They were rigid, relying on predefined rules that could not capture the complexity of evolving fraudulent activities.
Machine learning, on the other hand, offers a more dynamic approach. It uses algorithms that learn from historical transaction data, identifying patterns and anomalies that may indicate fraud. This ability to learn and adapt makes machine learning a powerful tool in detecting and predicting future frauds.
Moreover, machine learning can handle large volumes of data, making it ideal for the digital banking environment where millions of transactions occur daily.
Limitations of Conventional Systems in the Digital Age
In the digital age, the volume, velocity, and variety of transaction data have increased exponentially. Traditional fraud detection systems, designed for a less complex era, struggle to keep up.
These systems often generate a high number of false positives, flagging legitimate transactions as suspicious. This not only leads to unnecessary investigations but can also result in a poor customer experience.
Furthermore, conventional systems are reactive, often detecting fraud after it has occurred. In contrast, machine learning allows for proactive fraud detection, identifying potential fraud before it happens. This shift from a reactive to a proactive approach is crucial in minimising financial loss and protecting customer trust.
{{cta-first}}
Machine Learning: A Game Changer in Fraud Detection
Machine learning has emerged as a game changer in the field of fraud detection. Its ability to learn from data and adapt to new patterns makes it a powerful tool in the fight against financial fraud.
Machine learning algorithms can analyze vast amounts of transaction data in real-time. They can identify complex patterns and subtle correlations that may indicate fraudulent activity. This level of analysis is beyond the capabilities of traditional rule-based systems.
Moreover, machine learning can predict future frauds based on historical data. This predictive capability allows financial institutions to take proactive measures to prevent fraud, rather than reacting after the fact.
Machine learning also reduces the number of false positives. It can distinguish between legitimate transactions and suspicious activity with a high degree of accuracy. This not only saves resources but also improves the customer experience.
However, implementing machine learning in fraud detection is not without its challenges. It requires high-quality data, continuous model training, and a deep understanding of the underlying algorithms.
Understanding Machine Learning Algorithms in Banking
Machine learning algorithms can be broadly classified into supervised and unsupervised learning models. Supervised learning models are trained on labeled data, where the outcome of each transaction (fraudulent or legitimate) is known. These models learn to predict the outcome of new transactions based on this training.
Unsupervised learning models, on the other hand, do not require labeled data. They identify patterns and anomalies in the data, which can indicate potential fraud. These models are particularly useful in detecting new types of fraud that do not fit into known patterns.
Both supervised and unsupervised learning models have their strengths and weaknesses. The choice of model depends on the specific requirements of the financial institution and the nature of the data available.
Regardless of the type of model used, the effectiveness of machine learning in fraud detection depends largely on the quality of the data and the accuracy of the model training.
Real-Time Transaction Monitoring with Machine Learning
One of the key advantages of machine learning is its ability to process and analyse large volumes of data in real-time. This is particularly important in the context of digital banking, where transactions occur around the clock and across different channels.
Real-time transaction monitoring allows financial institutions to detect and prevent fraud as it happens. Machine learning algorithms can analyse each transaction as it occurs, flagging any suspicious activity for immediate investigation.
This real-time analysis is not limited to the transaction itself. Machine learning models can also analyze the context of the transaction, such as the customer's typical behavior, the time and location of the transaction, and other relevant factors.
This comprehensive analysis allows for more accurate fraud detection, reducing both false positives and false negatives. It also enables financial institutions to respond quickly to potential fraud, minimising financial loss and protecting customer trust.
Implementing Machine Learning Models for Fraud Detection
Implementing machine learning models for fraud detection requires a strategic approach. It's not just about choosing the right algorithms, but also about understanding the data and the business context.
The first step is to define the problem clearly. What type of fraud are you trying to detect? What are the characteristics of fraudulent transactions? What data is available for analysis? These questions will guide the choice of machine learning model and the design of the training process.
Next, the data needs to be prepared for analysis. This involves cleaning the data, handling missing values, and transforming variables as needed. The quality of the data is crucial for the performance of the machine learning model.
Once the data is ready, the machine learning model can be trained. This involves feeding the model with the training data and allowing it to learn from it. The model's performance should be evaluated and fine-tuned as necessary.
Finally, the model needs to be integrated into the existing fraud detection system. This requires careful planning and testing to ensure that the model works as expected and does not disrupt the existing processes.
Supervised vs. Unsupervised Learning in Fraud Detection
In the context of fraud detection, both supervised and unsupervised learning models have their uses. The choice between the two depends on the nature of the problem and the data available.
Supervised learning models are useful when there is a large amount of labeled data available. These models can learn from past examples of fraud and apply this knowledge to detect future frauds. However, they may not be as effective in detecting new types of fraud that do not fit into known patterns.
Unsupervised learning models, on the other hand, do not require labeled data. They can identify patterns and anomalies in the data, which can indicate potential fraud. These models are particularly useful in detecting new types of fraud that do not fit into known patterns.
Regardless of the type of model used, the effectiveness of machine learning in fraud detection depends largely on the quality of the data and the accuracy of the model training.
The Role of Data Quality and Model Training
Data quality plays a crucial role in the effectiveness of machine learning models for fraud detection. High-quality data allows the model to learn accurately and make reliable predictions.
Data quality involves several aspects, including accuracy, completeness, consistency, and timeliness. The data should accurately represent the transactions, be complete with no missing values, be consistent across different sources, and be up-to-date.
Model training is another critical factor in the success of machine learning for fraud detection. The model needs to be trained on a representative sample of the data, with a good balance between fraudulent and legitimate transactions.
The model's performance should be evaluated and fine-tuned as necessary. This involves adjusting the model's parameters, retraining the model, and validating its performance on a separate test set.
Continuous monitoring and updating of the model is also essential to ensure that it remains effective as new patterns of fraud emerge.
Challenges in Machine Learning-Based Fraud Detection
Despite the potential of machine learning in fraud detection, there are several challenges that financial institutions need to address. One of the main challenges is the complexity of financial transactions.
Financial transactions involve numerous variables and can follow complex patterns. This complexity can make it difficult for machine learning models to accurately identify fraudulent transactions.
Another challenge is the imbalance in the data. Fraudulent transactions are relatively rare compared to legitimate transactions. This imbalance can lead to models that are biased towards predicting transactions as legitimate, resulting in a high number of false negatives.
The dynamic nature of fraud is another challenge. Fraudsters continuously adapt their tactics to evade detection. This means that machine learning models need to be regularly updated to keep up with new patterns of fraud.
Finally, there are challenges related to data privacy and security. Financial transactions involve sensitive personal information. Financial institutions need to ensure that this data is handled securely and that privacy is maintained.
Distinguishing Legitimate Transactions from Fraudulent Activity
Distinguishing between legitimate transactions and fraudulent activity such as credit card fraud is a key challenge in fraud detection. This is particularly difficult because fraudulent transactions often mimic legitimate ones.
Machine learning models can help to address this challenge by identifying patterns and anomalies in the data. However, these models need to be trained on high-quality data and need to be regularly updated to keep up with changing patterns of fraud.
False positives are another concern. These occur when legitimate transactions are incorrectly flagged as fraudulent. This can lead to unnecessary investigations and can disrupt the customer experience. Strategies to minimise false positives include refining the model's parameters and incorporating feedback from fraud investigators.
Ethical and Privacy Considerations in Data Usage
The use of machine learning in fraud detection raises several ethical and privacy considerations. One of the main concerns is the use of personal transaction data.
Financial institutions need to ensure that they are complying with data protection regulations. This includes obtaining the necessary consents for data usage and ensuring that data is stored securely.
There is also a need for transparency in the use of machine learning. Customers should be informed about how their data is being used and how decisions are being made. This can help to build trust and can also provide customers with the opportunity to correct any inaccuracies in their data.
Finally, there are ethical considerations related to the potential for bias in machine learning models. Financial institutions need to ensure that their models are fair and do not discriminate against certain groups of customers. This requires careful design and testing of the models, as well as ongoing monitoring of their performance.
Financial Institutions Winning the Fight Against Fraud
Financial institutions are increasingly turning to machine learning to combat fraud. This is not just limited to large multinational banks. Smaller banks and credit unions are also adopting these technologies, often in partnership with fintech companies.
One example is the Royal Bank of Scotland, which uses machine learning to analyze customer behaviour and identify unusual patterns. This has helped the bank to detect and prevent fraud, improving customer trust and reducing financial loss.
Another example is Danske Bank, which uses machine learning to detect money laundering. The bank's machine learning model analyses transaction data and flags suspicious activity for further investigation. This has helped the bank to comply with anti-money laundering regulations and has also reduced the cost of investigations.
These examples show that machine learning is not just a tool for the future. It is already being used today, helping financial institutions to win the fight against fraud.
{{cta-ebook}}
The Future of Fraud Detection in Banking
The future of fraud detection in banking is promising, with machine learning playing a central role. As technology continues to evolve, so too will the methods used to detect and prevent fraud.
Machine learning models will become more sophisticated, capable of analysing larger volumes of data and identifying more complex patterns of fraudulent activity. This will enable financial institutions to detect fraud more quickly and accurately, reducing financial loss and improving customer trust.
At the same time, the integration of machine learning with other technologies, such as artificial intelligence and blockchain, will enhance fraud detection capabilities. These technologies will provide additional layers of security, making it even harder for fraudsters to succeed.
The future will also see greater collaboration between financial institutions, fintech companies, and law enforcement agencies. By sharing data and insights, these organizations can work together to combat financial fraud more effectively.
Emerging Trends and Technologies
Several emerging trends and technologies are set to shape the future of fraud detection in banking. One of these is deep learning, a subset of machine learning that uses neural networks to analyse data. Deep learning can identify complex patterns and correlations in data, making it a powerful tool for detecting fraud.
Another trend is the use of behavioural biometrics, which analyses the unique ways in which individuals interact with their devices. This can help to identify fraudulent activity, as fraudsters will interact with devices in different ways to legitimate users.
Finally, the use of consortium data and shared intelligence will become more common. By pooling data from multiple sources, financial institutions can build more accurate and robust machine learning models for fraud detection.
Preparing for the Next Wave of Financial Crimes
As technology evolves, so too do the methods used by fraudsters. Financial institutions must therefore be proactive in preparing for the next wave of financial crimes. This involves staying up-to-date with the latest trends and technologies in fraud detection, and continuously updating and refining machine learning models.
Financial crime investigators will also need to develop new skills and expertise. This includes understanding how machine learning works, and how it can be applied to detect and prevent fraud. Training and professional development will therefore be crucial.
Finally, financial institutions will need to adopt a multi-layered security approach. This involves using a range of technologies and methods to detect and prevent fraud, with machine learning being just one part of the solution. By doing so, they can ensure that they are well-prepared to combat the ever-evolving threat of financial fraud.
Conclusion: Embracing Machine Learning for a Safer Banking Environment
In conclusion, as financial institutions strive to stay ahead of increasingly sophisticated fraud tactics, adopting advanced solutions like Tookitaki's FinCense becomes imperative.
With its real-time fraud prevention capabilities, FinCense empowers banks and fintechs to screen customers and transactions with remarkable 90% accuracy, ensuring robust protection against fraudulent activities. Its comprehensive risk coverage, powered by cutting-edge AI and machine learning, addresses all potential risk scenarios, providing a holistic approach to fraud detection.
Moreover, FinCense's seamless integration with existing systems enhances operational efficiency, allowing compliance teams to concentrate on the most significant threats. By choosing Tookitaki's FinCense, financial institutions can safeguard their operations and foster a secure environment for their customers, paving the way for a future where fraud is effectively mitigated.
Experience the most intelligent AML and fraud prevention platform
Experience the most intelligent AML and fraud prevention platform
Experience the most intelligent AML and fraud prevention platform
Top AML Scenarios in ASEAN

The Role of AML Software in Compliance

The Role of AML Software in Compliance
